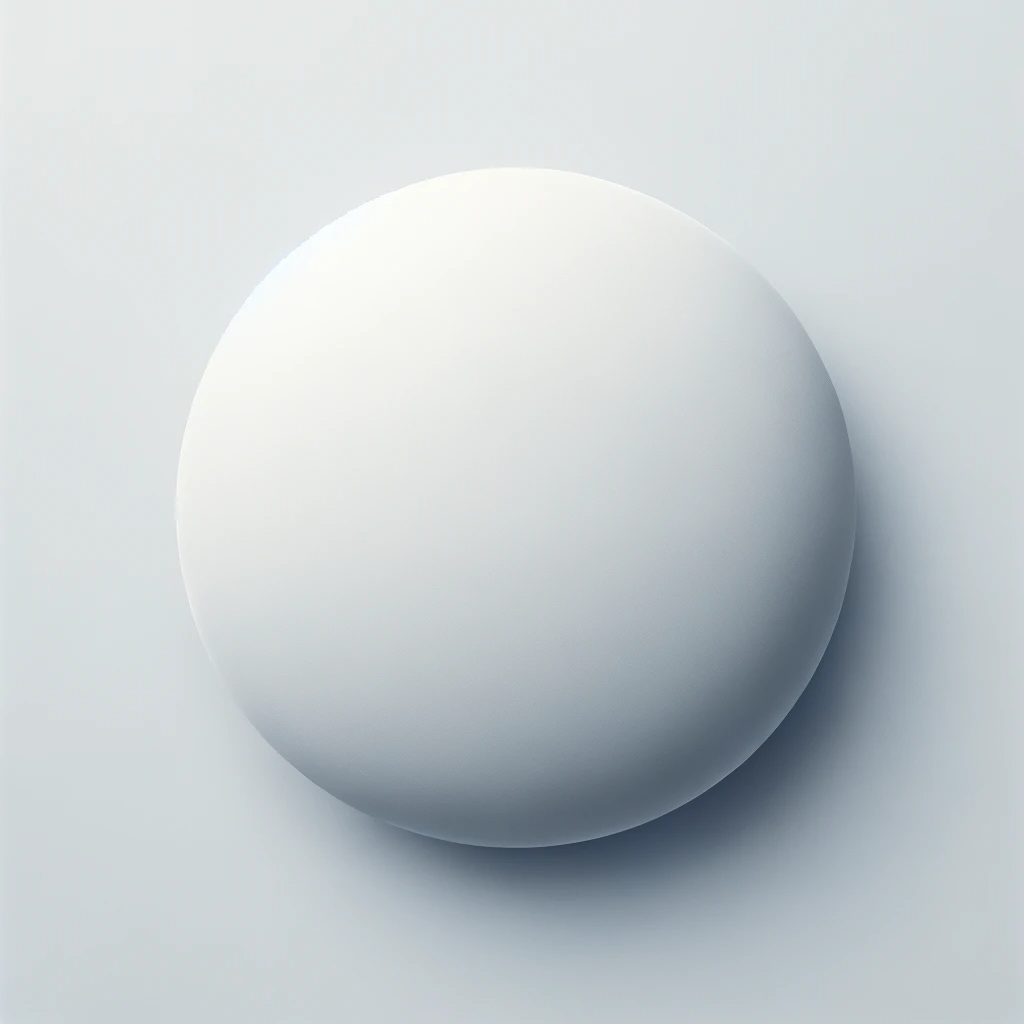
Unsupervised learning is a machine learning technique that analyzes and clusters unlabeled datasets without human intervention. Learn about the common unsupervised learning methods, such as clustering, association, and dimensionality reduction, and see examples of how they are used in data analysis and AI. For example, imagine a dataset of customers with information like age, income, and spending habits. Using K-means clustering, we could partition these customers ...This tutorial provides hands-on experience with the key concepts and implementation of K-Means clustering, a popular unsupervised learning algorithm, for customer segmentation and targeted advertising applications. By Abid Ali Awan, KDnuggets Assistant Editor on September 20, 2023 in Machine Learning. Image by Author.K means clustering in R Programming is an Unsupervised Non-linear algorithm that clusters data based on similarity or similar groups. It seeks to partition the observations into a pre-specified number of clusters. Segmentation of data takes place to assign each training example to a segment called a cluster.Download scientific diagram | 1: An example of (a) Supervised Learning (classification of cats and dogs) and (b) Unsupervised Learning (clustering of cats and dogs) from publication: Learning a ...Introduction. Supervised machine learning is a type of machine learning that learns the relationship between input and output. The inputs are known as features or ‘X variables’ and output is generally referred to as the …Download scientific diagram | 1: An example of (a) Supervised Learning (classification of cats and dogs) and (b) Unsupervised Learning (clustering of cats and dogs) from publication: Learning a ...Jan 3, 2023 · Supervised learning can be used to make accurate predictions using data, such as predicting a new home’s price. In order for predictions to be made, input data must be gathered. To determine a new home’s price, for example, we need to know factors like location, square footage, outdoor space, number of floors, number of rooms and more. Unsupervised machine learning is a fascinating field that enables data scientists and analysts to discover hidden patterns, group similar data, and reduce the dimensionality of complex datasets.Unsupervised learning is an increasingly popular approach to ML and AI. It involves algorithms that are trained on unlabeled data, allowing them to discover structure and relationships in the data. Henceforth, in this article, you will unfold the basics, pros and cons, common applications, types, and more about unsupervised learning.Feb 18, 2019 · An example of Unsupervised Learning is dimensionality reduction, where we condense the data into fewer features while retaining as much information as possible. An auto-encoder uses a neural ... Introduction. Clustering is an unsupervised machine learning technique with a lot of applications in the areas of pattern recognition, image analysis, customer analytics, market segmentation, social network analysis, and more. A broad range of industries use clustering, from airlines to healthcare and beyond. It is a type of unsupervised …The proposed model is an unsupervised building block for deep learning that combines the desirable properties of NADE and multi-prediction training: (1) its test likelihood can be computed analytically, (2) it is easy to generate independent samples from it, and (3) it uses an inference engine that is a superset of variational inference for …Competitive learning is a form of unsupervised learning in artificial neural networks, in which nodes compete for the right to respond to a subset of the input data. A variant of Hebbian learning, competitive learning works by increasing the specialization of each node in the network.It is well suited to finding clusters within data.. Models and … Unsupervised Learning. Unsupervised learning is about discovering general patterns in data. The most popular example is clustering or segmenting customers and users. This type of segmentation is generalizable and can be applied broadly, such as to documents, companies, and genes. Unsupervised learning consists of clustering models that learn ... Deep representation learning is a ubiquitous part of modern computer vision. While Euclidean space has been the de facto standard manifold for learning visual …Customer segmentation is a simple example of unsupervised learning. By leveraging an unsupervised learning approach, models can identify customer segments based on their behavior and preferences and help businesses to personalize their marketing strategies. Techniques and Algorithms. Unsupervised learning uses various methods, …What is the primary difference between supervised and unsupervised learning? A. Supervised learning requires labeled data, while unsupervised learning does not. B. Supervised learning is used for classification, while unsupervised learning is used for regression. C. Supervised learning is deterministic, while unsupervised learning is …Hello guys in this post we will discuss about Unsupervised Machine Learning Multiple Choice Questions and answers pdf.Unsupervised Machine Learning. All the notes which we are using are from taken geeksforgeeks. 1.In ________training model has only input parameter values. A) supervised learning. B) Unsupervised … The goal of unsupervised learning is to find the underlying structure of dataset, group that data according to similarities, and represent that dataset in a compressed format. Example: Suppose the unsupervised learning algorithm is given an input dataset containing images of different types of cats and dogs. The algorithm is never trained upon ... Semi-supervised learning is a machine learning method in which we have input data, and a fraction of input data is labeled as the output. It is a mix of supervised and unsupervised learning. Semi-supervised learning can be useful in cases where we have a small number of labeled data points to train the model.An example of this is the PCA and bivariate correlation analysis. By applying best subset regression iteratively over a number of variables, you can do a very complex sort of network estimation, as is assumed in structural equation modeling (strictly in the EFA sense). This, to me, seems like an unsupervised learning problem with regression.Feb 5, 2020 · What is an example of unsupervised learning in real life? An example of unsupervised learning in real life is customer segmentation in marketing. In this case, the algorithm analyzes customer data (purchase history, demographics, etc.) to identify distinct groups or segments based on similarities between customers. Unsupervised domain adaptive hashing is a highly promising research direction within the field of retrieval. It aims to transfer valuable insights from the source …Why it's important: We have tons of data, very few labels, and semi supervised learning is the only way to deal with that. Unsupervised learning is half of semisupervised learning. If it helps, you can think of it like using the unlabeled data to learn how to see, then the labeled data to learn the names of things.Example #3: Motion and Depth Estimation: a self-supervised learning technique used to predict motion and depth from video frames. This is an example of how self-supervised learning is used for training autonomous vehicles to navigate and avoid obstacles based on real-time video.What is the primary difference between supervised and unsupervised learning? A. Supervised learning requires labeled data, while unsupervised learning does not. B. Supervised learning is used for classification, while unsupervised learning is used for regression. C. Supervised learning is deterministic, while unsupervised learning is …Jul 27, 2022 ... ... machine learning model for you - supervised or Unsupervised learning? In this video, Martin Keen explains what the difference is between ...Feb 5, 2020 · What is an example of unsupervised learning in real life? An example of unsupervised learning in real life is customer segmentation in marketing. In this case, the algorithm analyzes customer data (purchase history, demographics, etc.) to identify distinct groups or segments based on similarities between customers. Aug 28, 2023 · 7 Unsupervised Machine Learning Real Life Examples k-means Clustering – Data Mining. k-means clustering is the central algorithm in unsupervised machine learning operations. It is the algorithm that defines the features present in the dataset and groups certain bits with common elements into clusters. Mar 19, 2021 · In supervised learning, a data scientist feeds the system with labeled data, for example, the images of cats labeled as cats, allowing it to learn by example. In unsupervised learning, a data scientist provides just the photos, and it's the system's responsibility to analyze the data and conclude whether they're the images of cats. Jul 31, 2019 · Introduction. Unsupervised learning is a set of statistical tools for scenarios in which there is only a set of features and no targets. Therefore, we cannot make predictions, since there are no associated responses to each observation. Instead, we are interested in finding an interesting way to visualize data or in discovering subgroups of ... Supervised learning is a process of providing input data as well as correct output data to the machine learning model. The aim of a supervised learning algorithm is to find a mapping function to map the input …K-Means Clustering is an Unsupervised Learning algorithm, which groups the unlabeled dataset into different clusters. Here K defines the number of pre-defined clusters that need to be created in the process, as if K=2, there will be two clusters, and for K=3, there will be three clusters, and so on.The results produced by the supervised method are more accurate and reliable in comparison to the results produced by the unsupervised techniques of machine learning. This is mainly because the input data in the supervised algorithm is well known and labeled. This is a key difference between supervised and unsupervised learning.Unsupervised learning is the machine learning task of ... Example of an unsupervised clustering algorithm.Magnitude, in astronomy, is a unit of measurement of the brightness of stars. Learn more and get a basic definition of magnitude at HowStuffWorks. Advertisement Magnitude, in astro...Customer and audience segmentation, computer vision and breach detection can all apply unsupervised learning. These two types of unsupervised learning methods are among the most common. Clustering Clustering algorithms are the most widely used example of unsupervised machine learning.1. What is unsupervised machine learning? 2. What are some real-life examples of unsupervised machine learning? 3. How does unsupervised machine learning differ …In some cases, it might not even be necessary to give pre-determined classifications to every instance of a problem if the agent can work out the classifications for itself. This would be an example of unsupervised learning in a classification context. Supervised learning is the most common technique for training neural networks and decision trees.1. What is unsupervised machine learning? 2. What are some real-life examples of unsupervised machine learning? 3. How does unsupervised machine learning differ …Supervised learning requires more human labor since someone (the supervisor) must label the training data and test the algorithm. Thus, there's a higher risk of human error, Unsupervised learning takes more computing power and time but is still less expensive than supervised learning since minimal human involvement is needed.Association rule learning is a type of unsupervised learning technique that checks for the dependency of one data item on another data item and maps accordingly so that it can be more profitable. ... We can understand it by taking an example of a supermarket, as in a supermarket, all products that are purchased together are put together. For ...Unsupervised Learning Clustering Algorithm Examples. Exclusive algorithms, also known as partitioning, allow data to be grouped so that a data point can belong to …8. First, two lines from wiki: "In computer science, semi-supervised learning is a class of machine learning techniques that make use of both labeled and unlabeled data for training - typically a small amount of labeled data with a large amount of unlabeled data. Semi-supervised learning falls between unsupervised learning (without any labeled ...Guitar legends make it look so easy but talent, skill, and perseverance are needed if you want to learn the guitar. There’s no definite age at which you should start learning the g...Example of an Anomalous Activity The Need for Anomaly Detection. According to a research by Domo published in June 2018, over 2.5 quintillion bytes of data were created every single day, and it was estimated that by 2020, close to 1.7MB of data would be created every second for every person on earth. And in times of CoViD-19, … Unsupervised learning is a machine learning technique that analyzes and clusters unlabeled datasets without human intervention. Learn about the common unsupervised learning methods, such as clustering, association, and dimensionality reduction, and see examples of how they are used in data analysis and AI. Aug 20, 2020 · Clustering. Cluster analysis, or clustering, is an unsupervised machine learning task. It involves automatically discovering natural grouping in data. Unlike supervised learning (like predictive modeling), clustering algorithms only interpret the input data and find natural groups or clusters in feature space. An example of this is the PCA and bivariate correlation analysis. By applying best subset regression iteratively over a number of variables, you can do a very complex sort of network estimation, as is assumed in structural equation modeling (strictly in the EFA sense). This, to me, seems like an unsupervised learning problem with regression.For example, an algorithm would be trained with pictures of dogs and other things, all labeled by humans, and the machine would learn ways to identify pictures of dogs on its own. Supervised machine learning is the most common type used today. In unsupervised machine learning, a programSupervised Learning. Supervised learning is a type of machine learning where the algorithm is trained on a labeled dataset. In this approach, the model is provided with …Unsupervised learning is a method in machine learning where, in contrast to supervised learning, algorithms learn patterns exclusively from unlabeled data.Unsupervised Learning Clustering Algorithm Examples. Exclusive algorithms, also known as partitioning, allow data to be grouped so that a data point can belong to …Mar 19, 2021 · In supervised learning, a data scientist feeds the system with labeled data, for example, the images of cats labeled as cats, allowing it to learn by example. In unsupervised learning, a data scientist provides just the photos, and it's the system's responsibility to analyze the data and conclude whether they're the images of cats. In the United States, no federal law exists setting an age at which children can stay home along unsupervised, although some states have certain restrictions on age for children to...Example #3: Motion and Depth Estimation: a self-supervised learning technique used to predict motion and depth from video frames. This is an example of how self-supervised learning is used for training autonomous vehicles to navigate and avoid obstacles based on real-time video.Deep representation learning is a ubiquitous part of modern computer vision. While Euclidean space has been the de facto standard manifold for learning visual …Example: One row of a dataset. An example contains one or more features and possibly a label. Label: Result of the feature. Preparing Data for Unsupervised Learning. For our …For example, an algorithm would be trained with pictures of dogs and other things, all labeled by humans, and the machine would learn ways to identify pictures of dogs on its own. Supervised machine learning is the most common type used today. In unsupervised machine learning, a programJan 3, 2023 · Unsupervised learning does not. Supervised learning is less versatile than unsupervised learning in that it requires the inputs and outputs of a data set to be labeled to provide a correct example for machine learning models to weigh predictions against. In other words, supervised learning requires human intervention to label data before the ... Semi-Supervised learning. Semi-supervised learning falls in-between supervised and unsupervised learning. Here, while training the model, the training dataset comprises of a small amount of labeled data and a large amount of unlabeled data. This can also be taken as an example for weak supervision.Semi-supervised learning is the type of machine learning that uses a combination of a small amount of labeled data and a large amount of unlabeled data to train models. This approach to machine learning is a combination of supervised machine learning, which uses labeled training data, and unsupervised learning, which uses unlabeled training …Mar 19, 2021 ... Examples of unsupervised machine learning · Anomaly detection: It's a process of finding atypical data points in datasets and, therefore, useful .....Semi-Supervised learning. Semi-supervised learning falls in-between supervised and unsupervised learning. Here, while training the model, the training dataset comprises of a small amount of labeled data and a large amount of unlabeled data. This can also be taken as an example for weak supervision.Supervised learning requires more human labor since someone (the supervisor) must label the training data and test the algorithm. Thus, there's a higher risk of human error, Unsupervised learning takes more computing power and time but is still less expensive than supervised learning since minimal human involvement is needed.Self-supervised learning is in some sense a type of unsupervised learning as it follows the criteria that no labels were given. However, instead of finding high-level patterns for clustering, self-supervised learning attempts to still solve tasks that are traditionally targeted by supervised learning (e.g., image classification) without any …Unsupervised Learning in Machine Learning (with Python Example) - JC Chouinard. 25 September 2023. Jean-Christophe Chouinard. Unsupervised learning is …Mar 19, 2021 · In supervised learning, a data scientist feeds the system with labeled data, for example, the images of cats labeled as cats, allowing it to learn by example. In unsupervised learning, a data scientist provides just the photos, and it's the system's responsibility to analyze the data and conclude whether they're the images of cats. It is a form of machine learning in which the algorithm is trained on labeled data to make predictions or decisions based on the data inputs.In supervised learning, the algorithm learns a mapping between the input and output data. This mapping is learned from a labeled dataset, which consists of pairs of input and output data.In Unsupervised Learning, you provide the model with unlabeled samples of data, give it time to find patterns and group those data samples together based on the patterns it arrives to. Technicalities The learning theory of Machine Learning models could fall under Supervised or Unsupervised Learning (or Reinforcement Learning in other …PyTorch Examples. This pages lists various PyTorch examples that you can use to learn and experiment with PyTorch. This example shows how to train a Vision Transformer from scratch on the CIFAR10 database. This …The learning algorithm can detect structure in the input information on its own. Simply put, Unsupervised Learning is a type of self-learning in which the algorithm can identify usually undiscovered patterns in unlabeled datasets and provide the appropriate output without intervention. Due to the lack of labels, unsupervised …Unsupervised Learning is a subfield of Machine Learning, focusing on the study of mechanizing the process of learning without feedback or labels. This is commonly understood as "learning structure". In this course we'll survey, compare and contrast various approaches to unsupervised learning that arose from difference disciplines, …K means clustering in R Programming is an Unsupervised Non-linear algorithm that clusters data based on similarity or similar groups. It seeks to partition the observations into a pre-specified number of clusters. Segmentation of data takes place to assign each training example to a segment called a cluster.For example, imagine a dataset of customers with information like age, income, and spending habits. Using K-means clustering, we could partition these customers ...Overview. Supervised Machine Learning is the way in which a model is trained with the help of labeled data, wherein the model learns to map the input to a particular output. Unsupervised Machine Learning is where a model is presented with unlabeled data, and the model is made to work on it without prior training and thus holds great potential ...This repository tries to provide unsupervised deep learning models with Pytorch - eelxpeng/UnsupervisedDeepLearning-Pytorch. ... The example usage can be found in test/test_vade-3layer.py, and it uses the pretrained weights from autoencoder in test/model/pretrained_vade-3layer.pt.Unsupervised Learning does not require the corresponding labels (y), the most common example of which being auto-encoders. Auto-encoders take x as input, pass it through a series of layers to compress the dimensionality and are then criticized on how well they can reconstruct x. Auto-encoders eventually learn a set of features that will ...K-means Clustering Algorithm. K-Means Clustering is an Unsupervised Learning algorithm. It arranges the unlabeled dataset into several clusters. Here K denotes the number of pre-defined groups. K can hold any random value, as if K=3, there will be three clusters, and for K=4, there will be four clusters.Generally, machine learning approaches used for anomaly detection can be categorized into supervised and unsupervised methods, with the presence of labels a key differentiator between the two. Lee et al. [ 10 ] developed an interpretable framework to visualize and process FOQA data and to identify safety anomalies in the data using …Member-only story. The Complete Guide to Unsupervised Learning. Understand principal component analysis (PCA) and clustering methods, and implement each algorithm in two mini projects. Marco …Example of Unsupervised Machine Learning. Let’s, take an example of Unsupervised Learning for a baby and her family dog. She knows and identifies this …Competitive learning is a form of unsupervised learning in artificial neural networks, in which nodes compete for the right to respond to a subset of the input data. A variant of Hebbian learning, competitive learning works by increasing the specialization of each node in the network.It is well suited to finding clusters within data.. Models and …. Association rule learning is a type of unAnother example of unsupervised machine learning is the The three machine learning types are supervised, unsupervised, and reinforcement learning. 1. Supervised learning. Gartner, a business consulting firm, predicts supervised learning will remain the most utilized machine learning among enterprise information technology leaders through 2022 [ 2 ].May 18, 2020 · Unsupervised learning is commonly used for finding meaningful patterns and groupings inherent in data, extracting generative features, and exploratory purposes. Examples of Unsupervised Learning. There are a few different types of unsupervised learning. We’ll review three common approaches below. Example: Finding customer segments In a nutshell, supervised learning is when a m Supervised learning is a machine learning task where an algorithm is trained to find patterns using a dataset. The supervised learning algorithm uses this training to make input-output inferences on future datasets. In the same way a teacher (supervisor) would give a student homework to learn and grow knowledge, supervised …1.6.2. Nearest Neighbors Classification¶. Neighbors-based classification is a type of instance-based learning or non-generalizing learning: it does not attempt to construct a general internal model, but simply stores instances of the training data.Classification is computed from a simple majority vote of the nearest neighbors of each point: a query … Feb 18, 2019 · An example of Unsupervised Learning is dimension...
Continue Reading