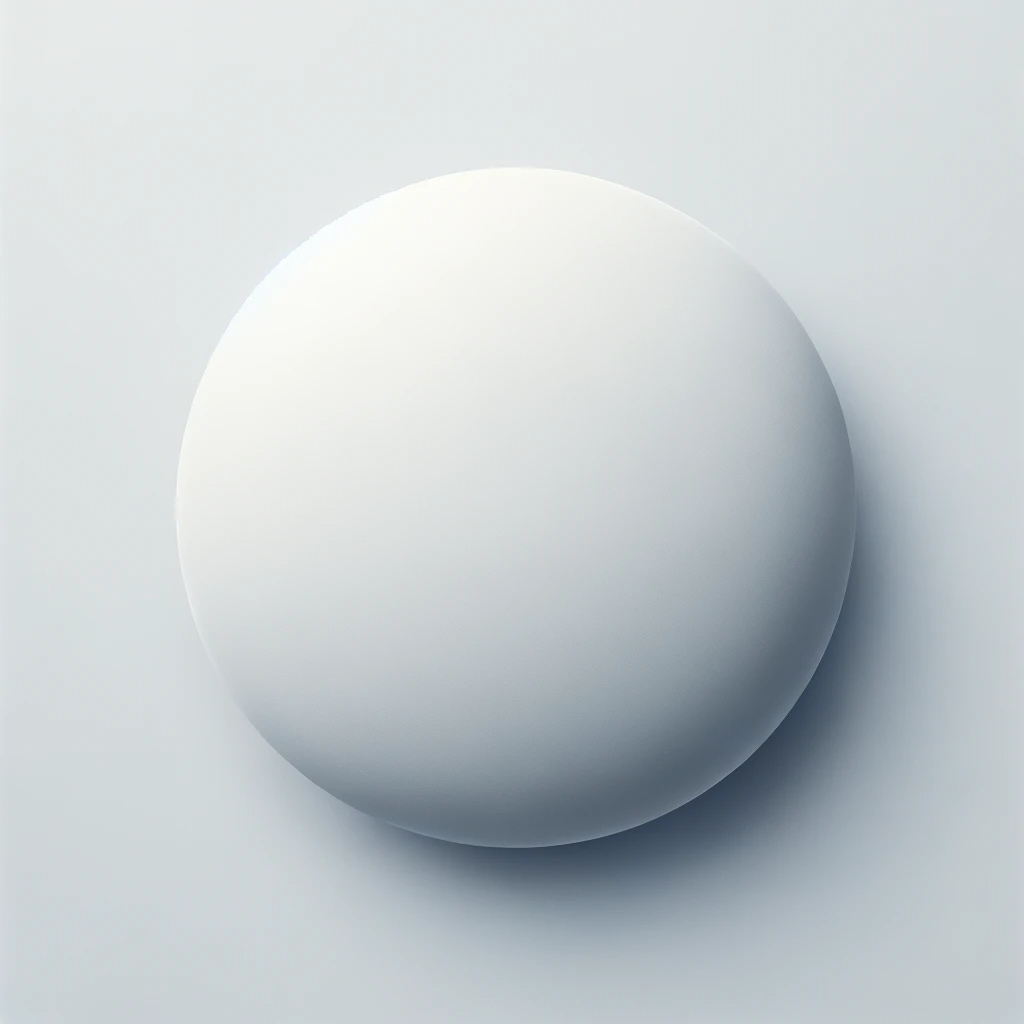
Step 3: Splitting the dataset into the Training set and Test set. Similar to the Decision Tree Regression Model, we will split the data set, we use test_size=0.05 which means that 5% of 500 data rows ( 25 rows) will only be used as test set and the remaining 475 rows will be used as training set for building the Random Forest Regression Model.Sep 5, 2018 ... Assumptions give you power - when they are valid. When the assumptions of a linear regression (or any other simple model) are fulfilled, ...Simple Linear Regression. Simple linear regression is useful for finding relationship between two continuous variables. One is predictor or independent variable and other is response or dependent variable. It looks for statistical relationship but not deterministic relationship. Relationship between two variables is said to be deterministic if ...May 20, 2020 · The Intuition behind Linear Regression. To many, Linear Regression is considered the “hello world” of machine learning.It is a fantastic starting point to highlight the capabilities of Machine Learning and the crossroads that exist between statistics and computer science. Linear regression models are simple but incredibly powerful; every introduction to machine learning should start here. The key principle of this method is that the impact of each predictor variable on the response variable can be specified with just a single number, which represents the ratio of change in the predictor to change in the …Classification is the task of predicting a discrete class label. Regression is the task of predicting a continuous quantity. There is some overlap between the algorithms for classification and regression; for example: A classification algorithm may predict a continuous value, but the continuous value is in the form of a probability for a class ... Logistic regression is another technique borrowed by machine learning from the field of statistics. It is the go-to method for binary classification problems (problems with two class values). In this post, you will discover the logistic regression algorithm for machine learning. After reading this post you will know: The many names and terms used when […] Mar 24, 2019 · Statistics vs Machine Learning — Linear Regression Example. I think this misconception is quite well encapsulated in this ostensibly witty 10-year challenge comparing statistics and machine learning. In Part One of this Bayesian Machine Learning project, we outlined our problem, performed a full exploratory data analysis, selected our features, and established benchmarks. Here we will implement Bayesian Linear Regression in Python to build a model. After we have trained our model, we will interpret the model parameters and use …Linear regression is a technique, while machine learning is a goal that can be achieved through different means and techniques. So regression performance is measured by how close it fits an expected line/curve, while machine learning is measured by how good it can solve a certain problem, with whatever means necessary. Figure 4. Graph of linear regression in problem 2. a) We use a table to calculate a and b. a) We first change the variable x into t such that t = x - 2005 and therefore t represents the number of years after 2005. Using t instead of x makes the numbers smaller and therefore manageable. The table of values becomes. 3. Linear Neural Networks for Regression¶. Before we worry about making our neural networks deep, it will be helpful to implement some shallow ones, for which ...Jun 26, 2018 ... Machine Learning Training with Python (Use Code "YOUTUBE20"): https://www.edureka.co/data-science-python-certification-course This ...Hence we need to find (m+1) variables denoted by beta_0, …,beta_m. It can be seen that linear regression is a special case of polynomial regression with degree 2. Consider the following set of data points plotted as a scatter plot. If we use linear regression, we get a fit that clearly fails to estimate the data points.In machine learning jargon the above can be stated as “It is a supervised machine learning algorithm that best fits the data which has the target variable ... You should find the appropriate value for the learning rate. Implementing Linear Regression in Scikit-Learn. Linear Regression with sklearn.Learn how to use linear regression, a fundamental concept in supervised learning, to predict a continuous outcome based on one or more predictor … The key ideas in linear regression are recycled everywhere, so understanding the algorithm is a must-have for a strong foundation in machine learning. Let's Be More Specific Linear regression is a supervised algorithm [ℹ] that learns to model a dependent variable, y y y , as a function of some independent variables (aka "features"), x i x_i x ... Jan 5, 2022 · Linear regression is a simple and common type of predictive analysis. Linear regression attempts to model the relationship between two (or more) variables by fitting a straight line to the data. Put simply, linear regression attempts to predict the value of one variable, based on the value of another (or multiple other variables). Are you a programmer looking to take your tech skills to the next level? If so, machine learning projects can be a great way to enhance your expertise in this rapidly growing field...1. In this project, we will see how to create a machine learning model that uses the Multiple Linear Regression algorithm. The main focus of this project is to explain how linear regression works, and how you can code a linear regression model from scratch using the awesome NumPy module. Of course, you can create a linear …Linear Regression :: Normalization (Vs) Standardization. I am using Linear regression to predict data. But, I am getting totally contrasting results when I Normalize (Vs) Standardize variables. Normalization = x -xmin/ xmax – xmin Zero Score Standardization = x …Linear regression is perhaps one of the most well known and well-understood algorithms in statistics and machine learning. Linear regression was developed in the field of statistics and is studied as a model for understanding the relationship between input and output numerical variables, but with the course of time, it has become an integral part of modern …Jan 24, 2019 ... In this video, Machine Learning in One Hour: Simple Linear Regression, Udemy instructors Kirill Eremenko & Hadelin de Ponteves will be ...For more information about Stanford’s Artificial Intelligence professional and graduate programs, visit: https://stanford.io/3pqkTryThis lecture covers super...The urine albumin–creatinine ratio (uACR) is a warning for the deterioration of renal function in type 2 diabetes (T2D). The early detection of ACR has become an important issue. Multiple linear regression (MLR) has traditionally been used to explore the relationships between risk factors and endpoints. Recently, machine learning (ML) …Jul 16, 2021 · Linear regression is a statistical method that tries to show a relationship between variables. It looks at different data points and plots a trend line. A simple example of linear regression is finding that the cost of repairing a piece of machinery increases with time. More precisely, linear regression is used to determine the character and ... The field of Data Science has progressed like nothing before. It incorporates so many different domains like Statistics, Linear Algebra, Machine Learning, ...Regression Summary §Supervised machine learning §Training data: Set of input values with numeric output value §Model is function from inputs to output Use function to predict output value for inputs §Balance complexity of function against “best fit” §Also useful for quantifying correlation For linear functions, the closer the function ... The key ideas in linear regression are recycled everywhere, so understanding the algorithm is a must-have for a strong foundation in machine learning. Let's Be More Specific Linear regression is a supervised algorithm [ℹ] that learns to model a dependent variable, y y y , as a function of some independent variables (aka "features"), x i x_i x ... Machine learning projects have become increasingly popular in recent years, as businesses and individuals alike recognize the potential of this powerful technology. However, gettin...Linear regression is the simplest machine learning model you can learn, yet there is so much depth that you'll be returning to it for years to come. That's why it's a great introductory course if you're interested in taking your first steps in the fields of: deep learning. machine learning. data science. statistics. In the first section, I will ...3. import torch. import numpy as np. import matplotlib.pyplot as plt. We will use synthetic data to train the linear regression model. We’ll initialize a variable X with values from − 5 to 5 and create a linear function that has a slope of − 5. Note that this function will be estimated by our trained model later. 1. 2.Are you a programmer looking to take your tech skills to the next level? If so, machine learning projects can be a great way to enhance your expertise in this rapidly growing field...If you’re itching to learn quilting, it helps to know the specialty supplies and tools that make the craft easier. One major tool, a quilting machine, is a helpful investment if yo... Simple Linear Regression. We will start with the most familiar linear regression, a straight-line fit to data. A straight-line fit is a model of the form: y = ax + b. where a is commonly known as the slope, and b is commonly known as the intercept. Consider the following data, which is scattered about a line with a slope of 2 and an intercept ... The Linear Regression Model. Before we begin the analysis, we'll examine the linear regression model to understand how it can help solve our problem. A linear …learning. In this lecture, we will select simple answers to these questions, leading to the linear regression framework. 3 Linear Regression ... Now that we have the linear regression framework set up, all that remains is to provide an algorithm to minimizetheMSE,L(w).Pokémon Platinum — an improved version of Pokémon Diamond and Pearl — was first released for the Nintendo DS in 2008, but the game remains popular today. Pokémon Platinum has many ...Skye, United Kingdom.Photo by Robert Lukeman on Unsplash. Boolean Dependent Variables, Probabilities & Odds. In this section we will explore the mathematics behind logistic regression, starting from the most basic model in machine learning—linear regression. In linear regression, the dependent variable d which is continuous and …Jun 16, 2022 ... Python is arguably the top language for AI, machine learning, and data science development. For deep learning (DL), leading frameworks like ...Linear Regression: Linear regression is a statistical regression method which is used for predictive analysis. It is one of the very simple and easy algorithms which works on regression and shows the relationship between the continuous variables. It is used for solving the regression problem in machine learning. In the simplestJan 5, 2022 · Linear regression is a simple and common type of predictive analysis. Linear regression attempts to model the relationship between two (or more) variables by fitting a straight line to the data. Put simply, linear regression attempts to predict the value of one variable, based on the value of another (or multiple other variables). Jan 8, 2021 ... datascience #linearregression #machinelearning #mlmodels Code - https://github.com/akmadan/ml_models_tutorial Telegram Channel- ...Mar 21, 2017 · Linear regression is a technique, while machine learning is a goal that can be achieved through different means and techniques. So regression performance is measured by how close it fits an expected line/curve, while machine learning is measured by how good it can solve a certain problem, with whatever means necessary. May 30, 2020 · Linear Regression is a machine learning (ML) algorithm for supervised learning – regression analysis. In regression tasks, we have a labeled training dataset of input variables (X) and a numerical output variable (y). Linear Regression Algorithm – Solved Numerical Example in Machine Learning by Mahesh HuddarThe following concepts are discussed:_____...In this tutorial, we assume you know the fundamentals of machine learning, including the basic concepts of linear regression. If you're not familiar with machine learning or are eager to refresh your machine learning skills, you might like to try our Data Scientist in Python Career Path .Chances are you had some prior exposure to machine learning and statistics. Basically, that’s all linear regression is — a simple statistics problem. Today you’ll learn the different types of linear regression and how to implement all of them in R: Introduction to Linear Regression; Simple Linear Regression from Scratch Figure 4. Graph of linear regression in problem 2. a) We use a table to calculate a and b. a) We first change the variable x into t such that t = x - 2005 and therefore t represents the number of years after 2005. Using t instead of x makes the numbers smaller and therefore manageable. The table of values becomes. The dataset a machine learning model uses to find a mathematical relationship between variables is called the training dataset. So, in order to build a linear regression model for our lemonade stand, we need to provide it with training data showing a correlation between temperature and profit margin. Take this sample training dataset, …Linear Regression. Linear Regression is one of the most important algorithms in machine learning. It is the statistical way of measuring the relationship between one or more independent variables vs one dependent variable. The Linear Regression model attempts to find the relationship between variables by finding the …Machine learning and data science have come a long way since being described as the “sexiest job of the 21st century” — we now have very powerful deep learning models capable of self driving automobiles, or seamlessly translating between different languages.Right at the foundation of all these powerful deep learning models is …Some of the benefits to science are that it allows researchers to learn new ideas that have practical applications; benefits of technology include the ability to create new machine...Linear Regression is a supervised machine learning algorithm. It tries to find out the best linear relationship that describes the data you have. It assumes that there exists a linear relationship between a dependent variable and independent variable (s). The value of the dependent variable of a linear regression model is a continuous value i.e ... Linear regression is one of the easiest and most popular Machine Learning algorithms. It is a statistical method that is used for predictive analysis. Linear regression makes predictions for continuous/real or numeric variables such as sales, salary, age, product price, etc. Linear regression algorithm shows a linear relationship between a ... For now, all you need to know is that it's an effective approach that can help you save lots of time when implementing linear regression under certain conditions. ... Andrew Ng, a prominent machine learning and AI expert, recommends you should consider using gradient descent when the number of features, n, is greater than 10,000.Machine Learning Cheat Sheet. In this cheat sheet, you'll have a guide around the top machine learning algorithms, their advantages and disadvantages, and use-cases. Apr 2022 · 8 min read. When working with machine learning, it's easy to try them all out without understanding what each model does, and when to use them.Dec 4, 2023 · The two main types of regression are linear regression and logistic regression. Linear regression is used to predict a continuous numerical outcome, while logistic regression is used to predict a binary categorical outcome (e.g., yes or no, pass or fail). 2. Through this course, you will learn how to: Evaluate a Linear Model. Select and Transform a Variable, as well as, Model Validation. Get an understanding of ‘Simple and Multiple Linear Regression.’. Deep dive into various nuances of building a robust predictive analytical solution with 6 hands-on data cases. Skill Type Emerging Tech.Introduction ¶. Linear Regression is a supervised machine learning algorithm where the predicted output is continuous and has a constant slope. It’s used to predict values within a continuous range, (e.g. sales, price) rather than trying to classify them into categories (e.g. cat, dog). There are two main types:Introduction ¶. Linear Regression is a supervised machine learning algorithm where the predicted output is continuous and has a constant slope. It’s used to predict values within a continuous range, (e.g. sales, price) rather than trying to classify them into categories (e.g. cat, dog). There are two main types:Next, let's begin building our linear regression model. Building a Machine Learning Linear Regression Model. The first thing we need to do is split our data into an x-array (which contains the data that we will use to make predictions) and a y-array (which contains the data that we are trying to predict. First, we should decide which columns to ...The Linear Regression Model. Before we begin the analysis, we'll examine the linear regression model to understand how it can help solve our problem. A linear …2.1. (Regularized) Logistic Regression. Logistic regression is the classification counterpart to linear regression. Predictions are mapped to be between 0 and 1 through the logistic function, which means that predictions can be interpreted as class probabilities.. The models themselves are still “linear,” so they work well when your classes are …Machine Learning-Linear regression. Sep 23, 2019 •. 4 likes • 4,672 views. K. kishanthkumaar Follow. Linear Regression is one of the basic and fundamental algorithm which is used in machine learning. Data & Analytics. 1 of 10. Download Now.IMO, deep learning is under the machine learning umbrella, in that it is deep machine learning, instead of "shallow" machine learning methods (e.g., OLS, KNN, SVM, Random Forest). Deep learning and artificial neural networks can be used for regression problems, to add another OLS alternative path for you.Linear Regression is a supervised learning algorithm which is generally used when the value to be predicted is of discrete or quantitative nature. It tries to establish a relationship between the dependent variable ‘y’, and one or more related independent variables ‘x’ using what is referred to as the best-fit line.For now, all you need to know is that it's an effective approach that can help you save lots of time when implementing linear regression under certain conditions. ... Andrew Ng, a prominent machine learning and AI expert, recommends you should consider using gradient descent when the number of features, n, is greater than 10,000.Some of the benefits to science are that it allows researchers to learn new ideas that have practical applications; benefits of technology include the ability to create new machine...Apr 17, 2020 · For more information about Stanford’s Artificial Intelligence professional and graduate programs, visit: https://stanford.io/3pqkTryThis lecture covers super... Skye, United Kingdom.Photo by Robert Lukeman on Unsplash. Boolean Dependent Variables, Probabilities & Odds. In this section we will explore the mathematics behind logistic regression, starting from the most basic model in machine learning—linear regression. In linear regression, the dependent variable d which is continuous and …Three linear machine learning algorithms: Linear Regression, Logistic Regression and Linear Discriminant Analysis. Five nonlinear algorithms: Classification and Regression Trees, Naive Bayes, K-Nearest Neighbors, Learning Vector Quantization and Support Vector Machines. Can someone please explain for each of these algorithms …Simple linear regression is a type of regression analysis where the number of independent variables is one and there is a linear relationship between the …Step 4: Testing the Linear Regressor. To test the regressor, we need to use it to predict on our test data. We can use our model’s .predictmethod to do this. predictions = regressor.predict(x_test) Now the …Machine learning algorithms are at the heart of predictive analytics. These algorithms enable computers to learn from data and make accurate predictions or decisions without being ...In machine learning, support vector machines (SVMs, also support vector networks) are supervised max-margin models with associated learning algorithms that analyze data for …Learn how linear regression works on a fundamental level and how to implement it from scratch or with scikit-learn in Python. Find out the main idea, the … Understanding linear regression. Let’s understand what linear regression is all about from a non-technical perspective, before we get into the details, we will first understand from a layman’s terms what linear regression is. Now, linear regression is a machine learning algorithm ml algorithm that uses data to predict a quantity of interest ... Jan 21, 2021 ... Advantages of Linear Regression · The implementation of Linear regression is very simple. · Linear regression fits linearly separable datasets ....Regression methods are then discussed with fair length focusing on linear regression. We conclude the research with an application of a real-life regression problem. Example of association learning. Machine Learning-Linear regression. Sep 23, 20The linear regression model comprising gradient desc In statistics and machine learning, a loss function quantifies the losses generated by the errors that we commit when: we estimate the parameters of a statistical model; we use a predictive model, such as a linear … Linear regression is a supervised learning algori Machine Learning Algorithms for Regression (original image from my website). In my previous post “Top Machine Learning Algorithms for Classification”, we walked through common classification algorithms. Now let’s dive into the other category of supervised learning — regression, where the output variable is continuous and numeric.Hence we need to find (m+1) variables denoted by beta_0, …,beta_m. It can be seen that linear regression is a special case of polynomial regression with degree 2. Consider the following set of data points plotted as a scatter plot. If we use linear regression, we get a fit that clearly fails to estimate the data points. Linear regression is one of the easiest and most...
Continue Reading